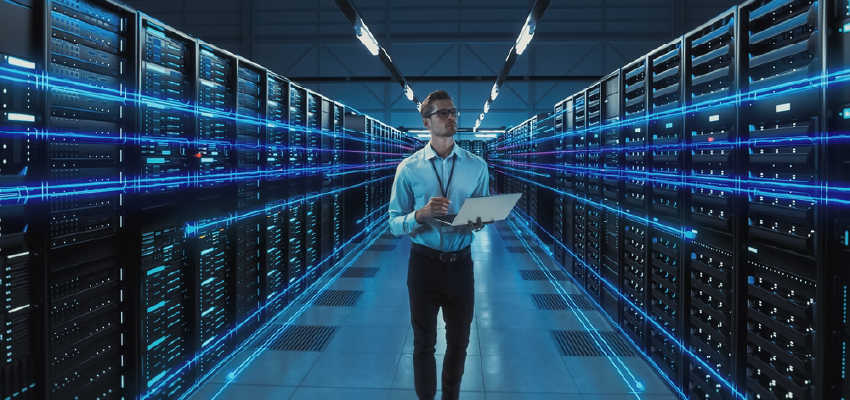
In the realm of modern business operations, data engineering services have emerged as a pivotal asset driving strategic decisions and operational efficiencies. Effective management and utilization of data require robust data engineering practices, particularly in optimizing data pipelines for peak performance. According to Gartner, by 2023, over 50% of businesses will adopt data engineering as a primary focus to enhance their analytics capabilities. Here, we will delve into key strategies and best practices essential for enhancing data pipeline efficiency.
Understanding Data Engineering
Data engineering involves designing, building, and maintaining data pipelines that collect, transform, and transport data from various sources to storage and analytics systems. It encompasses the tools, techniques, and processes required to handle big data and ensure its accessibility and reliability.
Data engineering services involve various tasks to handle and convert data into a form that can be analyzed effectively. These services include data pipeline development, data integration, data warehousing, and more. Organizations often leverage data engineering as a service (DEaaS) to streamline these processes and improve overall data management efficiency. Stat: The global data engineering market is projected to grow at a CAGR of 17.6% from 2021 to 2028, reaching $86.9 billion by 2028 (Fortune Business Insights).
Importance of Data Engineering in Service Delivery
Data engineering as a service enables organizations to harness the expertise of specialized providers who offer tailored solutions for data pipeline optimization. By partnering with data engineering consulting services, businesses can benefit from customized strategies that align with their specific needs and objectives. These consultants act as strategic partners, helping organizations navigate complex data landscapes and implement efficient data management practices.
Data engineering services are the backbone of any successful data strategy. These services encompass the design, development, and maintenance of data infrastructure, including data pipelines, data warehouses, and data lakes. By leveraging data engineering services, organizations can ensure that their data is reliable, scalable, and accessible, enabling them to make informed decisions and drive business growth. Fact: Companies with mature data strategies are 70% more likely to have an in-house or partnered data engineering team (IDC).
Importance of Optimizing Data Pipelines
Optimizing data pipelines is critical for several reasons:
Efficiency: Improved pipeline performance reduces latency in data processing, enabling faster decision-making and responsiveness. Stat: 90% of data leaders report that improving data pipeline efficiency has directly contributed to better decision-making (Forrester).
Cost-Effectiveness: Efficient pipelines utilize resources more effectively, minimizing cloud infrastructure costs. Fact: Organizations that optimize their data pipelines can reduce cloud costs by up to 40% (McKinsey).
Scalability: Well-optimized pipelines can handle growing volumes of data without significant performance degradation. Stat: 85% of organizations that prioritize data pipeline scalability see improved data processing speed by over 30% (Gartner).
Reliability: Reliable pipelines ensure data integrity and consistency, crucial for accurate analytics and reporting. Fact: Data quality issues cost businesses an average of $15 million per year (IBM).
Best Practices for Optimizing Data Pipelines
Use of Efficient Data Storage and Processing Technologies Selecting appropriate technologies such as cloud-based data warehouses (e.g., Amazon Redshift, Google BigQuery) and distributed computing frameworks (e.g., Apache Spark, Hadoop) can significantly enhance pipeline performance. These technologies offer scalability and parallel processing capabilities essential for handling large datasets efficiently. Stat: Companies using cloud-based data storage and processing report a 40% increase in data accessibility (Deloitte).
Data Partitioning and Indexing Partitioning data based on key attributes (e.g., date, region) and creating indexes can expedite data retrieval and aggregation processes. This practice reduces the amount of data scanned per query, thereby improving overall pipeline performance. Automated tools and frameworks often support partitioning and indexing for various data formats. Fact: Proper data partitioning can reduce query times by up to 90% (Amazon Web Services).
Optimized Data Transformations Efficient data transformations involve minimizing unnecessary computations and leveraging batch or streaming processing based on the use case. Technologies like Apache Beam or Apache Flink facilitate complex transformations while ensuring high throughput and low latency. Stat: Streamlined data transformations can increase data processing efficiency by up to 25% (TechRepublic).
Monitoring and Performance Tuning Continuous monitoring of pipeline performance metrics (e.g., throughput, latency, error rates) is essential for identifying bottlenecks and inefficiencies. Implementing automated alerting mechanisms enables proactive resolution of issues, ensuring consistent pipeline performance. Fact: 75% of organizations with robust monitoring strategies see a 50% reduction in pipeline downtimes (Gartner).
Data Quality Assurance Keeping data quality high at every step of the process is essential to get accurate insights. Implementing data validation checks, error handling mechanisms, and schema evolution strategies ensures that only high-quality data enters the analytics process. Data engineering consulting services often specialize in implementing robust data quality frameworks. Stat: Companies that focus on data quality assurance experience 60% fewer errors in their analytics outputs (Harvard Business Review).
Leveraging Data Engineering Service Providers
Data engineering service providers offer specialized expertise in optimizing data pipelines tailored to specific industry requirements. These providers collaborate closely with businesses to understand their data ecosystem, design scalable architectures, and deploy efficient data processing solutions. By partnering with established data engineering service providers, organizations can accelerate time-to-insight and gain a competitive edge through advanced analytics capabilities. Fact: Organizations that partner with data engineering service providers report a 35% faster time-to-insight (Deloitte).
Building and maintaining a high-performance data pipeline requires specialized skills and expertise. Data engineering service providers possess the knowledge and experience to design, develop, and manage your data pipelines effectively. Partnering with these experts offers several benefits:
Reduced Costs: Building and maintaining an in-house data engineering team can be expensive. Data engineering as a service provides a cost-effective alternative, allowing you to access skilled professionals without significant upfront investment. Stat: Companies using DEaaS save an average of 25% on operational costs (Forrester).
Faster Time to Value: Data engineering service providers can help you get your data pipelines up and running quickly, accelerating your time to valuable insights. Fact: Businesses with optimized data pipelines experience a 40% reduction in time-to-market for new products (McKinsey).
Access to Expertise: These service providers bring a wealth of experience and best practices to the table, ensuring your data pipelines are built with performance and scalability in mind. Stat: 80% of companies with dedicated data engineering partners report higher satisfaction with their data infrastructure (Gartner).
Conclusion
Optimizing data pipelines for performance is a continuous journey that requires a combination of technical expertise, strategic planning, and innovative tools. By adopting best practices such as efficient technology selection, data partitioning, and continuous monitoring, organizations can enhance their data engineering capabilities and derive greater value from their data assets. Leveraging data engineering consulting services and data engineering as a service can further streamline these efforts, ensuring scalable and efficient data pipelines that drive business success.
In summary, data engineering best practices are essential for maximizing the value of data through efficient pipeline optimization and management. Whether through data engineering as a service or consulting, prioritizing these practices ensures that data pipelines remain robust, reliable, and aligned with business objectives.